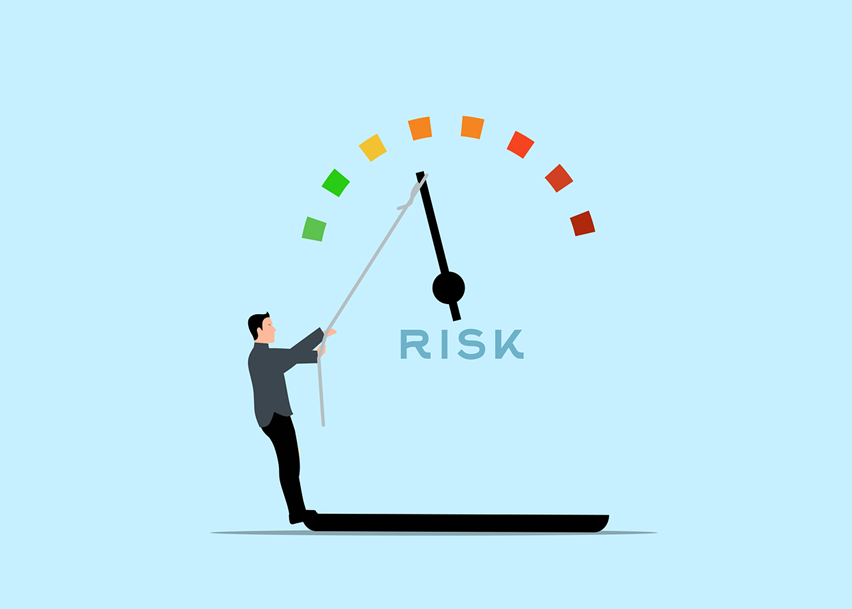
Today’s volatile economy, combined with operational risks and extensive local and international regulations, complicate the work of risk managers. However, modern technologies such as Artificial intelligence (AI) and Machine Learning (ML) have come in handy.
They offer an efficient way to identify and control risks, thus safeguarding themselves and their customers against threats.
What Is Risk Management?
Businesses can’t operate without risks. Technological, environmental, economic, and competitive factors introduce barriers that organisations must not only manage but also overcome.
According to PwC’s Global Risk Survey, companies implementing strategic risk management are five times more likely to increase stakeholder confidence and improve business outcomes. They are also two times more likely to realise faster revenue growth.
But what exactly is risk management? It is a systematic process of assessing, identifying, and mitigating uncertainties or threats affecting an organisation. In other words, it involves the following:
- Analysing the possibility of risks occurring
- Accessing its likely impact
- Creating strategies to minimise harm
- Monitoring measures’ effectiveness
Why Is Risk Management Important?
There are several reasons why risk management is important. Mainly, it’s because it:
- Protects organisation’s reputation: In most instances, effective risk management proactively safeguards an organisation from happenings that can affect its reputation.
- Minimises losses: Most organisations create risk management departments to avoid major financial losses.
- Encourages innovation and growth: Risk management is more than just preventing negative occurrences. It could also be a spark that ignites the creativity and growth of an organisation.
- Enhances decision-making: It provides a structured framework for decision-making.
What’s the Difference Between AI and ML?
AI is computer software that simulates the way humans think. That way, it is able to carry out complex tasks such as reasoning, learning, and analysing.
Machine learning is a key subset of AI that employs algorithms trained on data to come up with models that can perform these complex tasks.
Most AI is performed using ML, so the two terms are frequently used synonymously. However, AI refers to the general notion of developing human-like cognition using computer software, whereas ML is only one way of doing so.
Key Benefits of AI and ML in Risk Management
The use of AI and ML for risk management comes with multiple benefits. Check out more about them below.
Greater Efficiency
Gathering, grouping, and processing data used to be a time- and labour-intensive process. AI and ML can handle large volumes of data using fewer resources.
Improved Timelessness
The ideal time to know about a certain risk is now. AI and machine learning allow real-time monitoring so we can identify and react to risks more quickly than was possible before.
Improved Data Analysis and Pattern Recognition
A big portion of all data generated is unstructured. Yet, the most crucial information is often hidden in unstructured data.
In risk management, organisations need to be able to deal with both known and not-so-easily visible risks. AI and ML are good at quickly analysing unstructured data and developing actionable insights from it.
Use Cases and Applications of AI in Risk Management
The most common use cases and applications of AI supporting risk management processes include the following aspects you can read about in the sections below.
Fraud Detection
For financial services firms, fraud detection requires a vast amount of diverse data and intensive processing.
Machine learning engines and AI systems can help detect fraudulent activities and transactions by assisting with database searches, text mining, social network analysis, and anomaly detection techniques. This could also be extended to fraudulent use of cloud services – for example, a phishing attack from a hacked Microsoft 365 account.
Credit Scoring and Risk Assessment for Loan Disbursements
AI has become a game-changer in determining credit scores and risk assessment for loan disbursements.
AI systems scrutinise a wealth of data, including savings patterns, transaction histories, and other financial behaviours. For example, an AI might look at an individual’s cash flow stability, focusing on consistent savings as an indicator of financial health. In addition, AI can use non-conventional data, such as online purchasing behaviours or payment histories, offering a complete picture of creditworthiness.
This depth of analysis allows lenders to spot responsible borrowers whom traditional scoring models would have underserved. By capturing these variables, AI and ML helps banks mitigate risks and make sound decisions about loan approvals.
AI to Promote Responsible Gambling in Online Casinos
AI’s capabilities extend to promoting responsible gambling in online casinos. Let’s say you have won some free spins no deposit bonuses at Gamblenator (an Australian resource for everything casino promotions).
Normally, these free spins provide extra chances to win without risking your money. However, a few players may feel less cautious when using spins as they didn’t directly pay for them. This may lead to decisions founded on the excitement of the moment rather than rational judgement. Here, AI steps in as a proactive tool for player protection.
AI can pick out signs of problem gambling, like erratic betting patterns or chasing losses. Operators can then intervene, imposing limits or offering relevant resources to help players manage their gambling habits. Eventually, this protects players and improves the reputation of an online casino as a responsible operator.
Threat Intelligence Analysis
ML can extract crucial insights from data on the likelihood of threat or risk occurring.
For instance, machinery in areas like mining and manufacturing is usually monitored by multiple sensors. AI and machine learning can recognise patterns linked with malfunction to allow for an accurate evaluation of equipment health and proactive maintenance to prevent breakdown.
Workplace Risk Reduction
One of the common types of risks in organisations involves workers. Al and ML models can analyse and process data related to workplace activities in high-risk settings, where accidents can be hazardous or even fatal.
AL algorithms can assess behavioural patterns identified before accidents occur and develop predictive scenarios to help improve safety protocols and prevent accidents.
Also, they can help manage other kinds of people’s risks, such as identifying unethical or illegal behaviours by workers by analysing emails and other communications.
Risk Management in Insurance
AI-powered risk management has positively changed the insurance industry by improving underwriting and claims processing.
Aspect | Description |
Underwriting | AI enhances risk assessment by analysing vast datasets and identifying trends indicative of higher risk profiles. This enables informed underwriting decisions based on factors such as vehicle type, driving history, and social data. |
Claims processing | AI expedites the assessment of damages through image recognition, facilitating faster settlements for both policyholders and insurers. |
Fraud detection | AI reduces fraudulent claims by detecting anomalies in claim histories or submitted documents, mitigating potential losses for insurers. |
Personalisation | Insurers can offer competitive premiums and tailored policies based on individual risk profiles, enhancing customer satisfaction and setting new industry standards. |
Market Risk Analysis
Analysts can predict market uncertainties more accurately using AI and ML in risk management. AI algorithms process massive market data, detecting even the smallest patterns humans may miss.
For instance, AI can analyse social media trends to predict market shifts. This can notify investors about a potential opportunity or downturn, influencing trading strategies. With AI-powered risk management, organisations can respond to market changes quickly, reducing potential losses.
Investment firms can also gain a competitive advantage from AI’s insights. They can anticipate risks and adjust portfolio strategies accordingly. Essentially, AI and ML are central in nearly all modern financial risk management efforts.
Cybersecurity Threat Detection
The application of AI in risk management is also helpful in detecting cybersecurity threats. Artificial intelligence systems are trained to track network traffic and identify unusual patterns that may point to a breach. For example, an AI system might detect several unsuccessful login attempts from a foreign IP address. This may signal a potential security threat.
With AI, organisations can quickly neutralise such threats. The AI systems automatically activate defensive protocols, such as blocking the wary IP addresses. This response is crucial in thwarting data breaches or further infiltration.
AI tools are also good at spotting ransomware and malware signatures. They cross-reference network activities against known threat databases for immediate identification. Such proactive monitoring is necessary to maintain cybersecurity in a constantly changing threat landscape.
Regulatory Compliance Management
Complying with international and local regulations is a very important and complex activity, especially in the financial industry. Huge data sets must be reviewed, countless variables need to be analysed, and accurate reports should be submitted to the relevant authorities.
AI and ML, automates these activities and ensures they are completed accurately and efficiently. This allows financial institutions to avoid fines and penalties, which results in considerable cost savings.
ML can also help cut the number of false alerts in the compliance system. This ensures issues are handled manually by a human only when necessary.
Final Thoughts
The future of AI and ML in risk management looks bright. As technology advances, AI and ML will be capable of becoming more predictive, refined, and incorporated across multiple industrial domains. Also, with further innovations, organisations can expect more complex AI models that offer deeper insight and more accurate risk evaluation.